
The people of Mecca were descended from nomads, only a generation or two back, and still retained much of the nomadic outlook and practice. Islam did not origi nate in the desert, however, but in the small urban community of Mecca, which by the early seventh century was an important commercial center and distinctly prosperous. l The majority of the inhabitants of Arabia were nomads, organized in clans and tribes, and wresting a living from a difficult environ ment by pasturing camels and other animals.
SCONTENT FMAN MANUAL
We show that the automatic ver-bosity scores are significantly negatively correlated with manual content quality scores given to the summaries.Before embarking on a discussion of the attitudes toward warfare found in the Islamic religion, it is necessary to say something about conditions in Arabia immediately before the appearance of Islam. We use data from manual evaluation of summarization systems to assess the verbosity scores produced by our model. During test time, the difference between actual and predicted length allows us to quantify text verbosity. Weights for these features are learned using a corpus of summaries written by experts and on high quality journalistic writing. including syntactic phrasing, constituent compression probability, presence of named entities, sentence specificity and inter-sentence continuity. We consider a range of features to approximate content type.
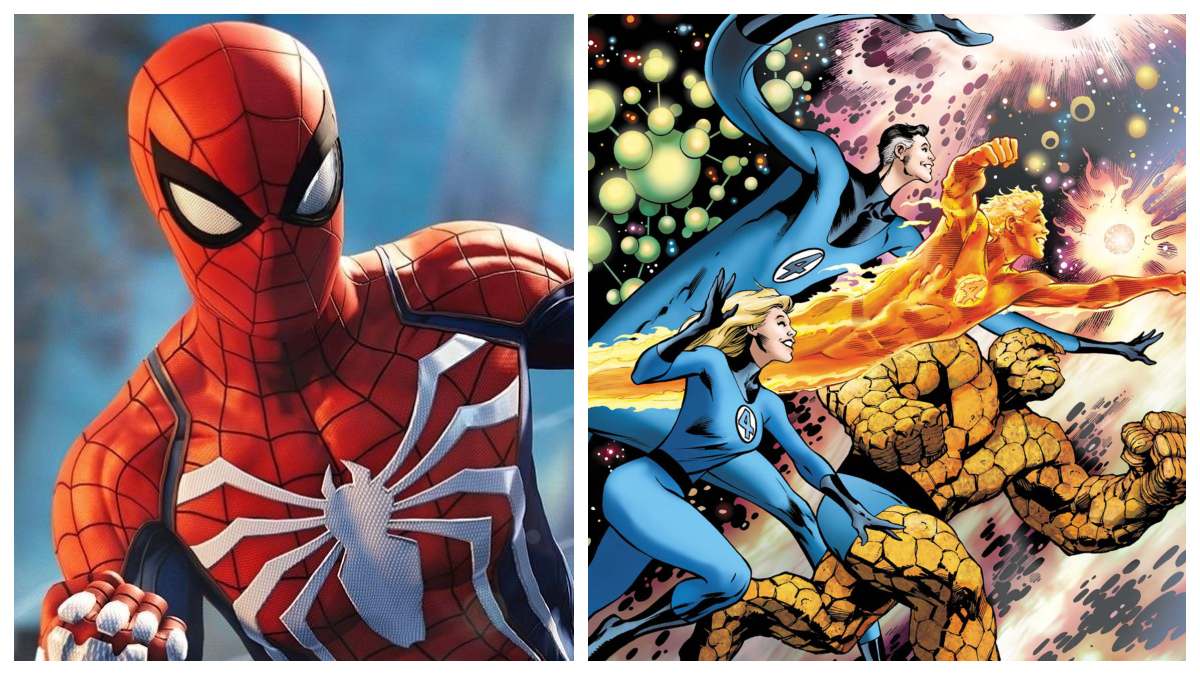
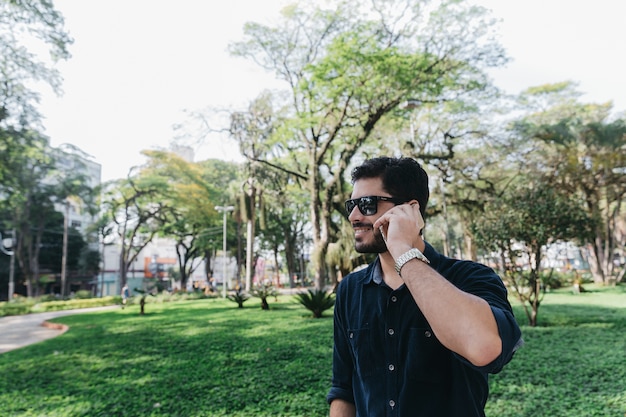
Specifically, our model predicts the appropriate length for texts based on content types present in a snippet of constant length. Here we propose the first model to computationally assess if a text deviates from these requirements. Length constraints impose implicit requirements on the type of content that can be included in a text. This method of comparison with the consensus of systems produces impressively accurate rankings of system summaries, achieving correlation with human rankings above 0.9. Finally, we explore the feasibility of another measure-similarity between a system summary and the pool of all other system summaries for the same input. Combining the pseudomodels with the single human model to form the gold-standard leads to higher correlations with human judgments compared to using only the one available model. We introduce pseudomodels, which are system summaries deemed to contain good content according to automatic evaluation. We also explore ways of increasing evaluation quality when only one human model summary is available as a gold standard. We show that quantifying the similarity between the source text and its summary with appropriately chosen measures produces summary scores which replicate human assessments accurately. The third technique improves standard automatic evaluations by expanding the set of available model summaries with chosen system summaries. are model-free and do not rely on a gold standard for the assessment. We propose three novel evaluation techniques. This evaluation paradigm falls short when human summaries are not available and becomes less accurate when only a single model is available. The most widely adopted approaches for evaluation of summary content follow some protocol for comparing a summary with gold-standard human summaries, which are traditionally called model summaries. For consistency and fluency-qualities focused on local text details-the most useful layers are close to the top (but not at the top) for coherence and relevance we found a more complicated and interesting picture.
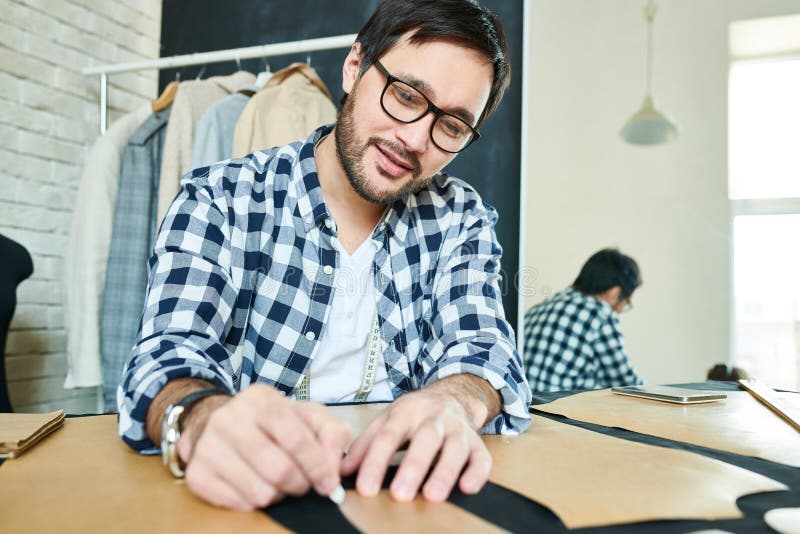
We observe that useful information exists in almost all of the layers except the several lowest ones.
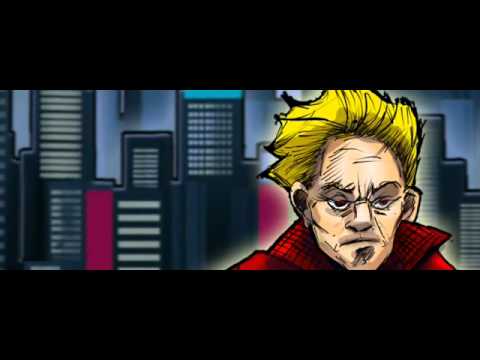
As ESTIME uses points of contextual similarity, it provides insights into usefulness of information taken from different BERT layers. In this work we generalize the method, making it applicable to any text-summary pairs. This is not a problem for current styles of summarization, but it may become an obstacle for future summarization systems, or for evaluating arbitrary claims against the text. The ESTIME measure, recently proposed specifically for factual consistency, achieves high correlations with human expert scores both for consistency and fluency, while in principle being restricted to evaluating such text-summary pairs that have high dictionary overlap. Factual consistency is one of important summary evaluation dimensions, especially as summary generation becomes more fluent and coherent.
